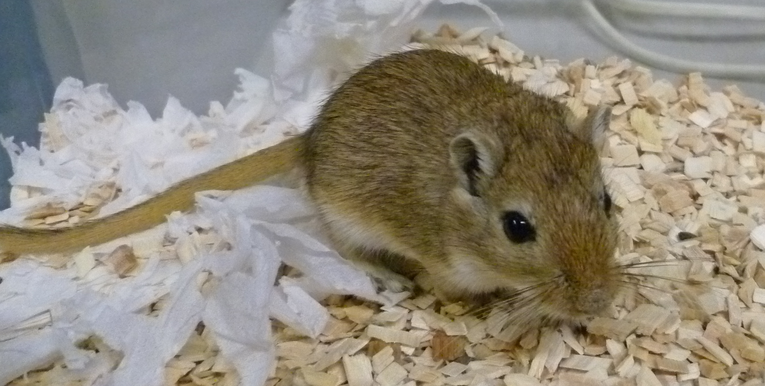
Neurophysiology of hearing: Hearing loss, tinnitus and auditory learning
Our standard animal model is the Mongolian gerbil (Meriones unguiculatus); iit is a widely used model in auditory research as it has a human-like hearing range. The organization of the auditory system of these animals is well described; therefore, they are perfectly suited to investigate pathological neuro-plastic changes of that system, e.g., after acoustic noise trauma induced hearing loss or a resulting tinnitus percept development. We investigate the neurophysiological mechanisms of such pathological changes and correlate them with behavioral tests for hearing or for the existence of a tinnitus percept. Our aim is to allow the develop of new therapeutical approaches by a deeper understanding of these patho-mechanisms. In cooperation with the audiology (Prof. Hoppe) of our hospital we are able to test our acquired knowledge directly on the patients.
Physiology and Pathophysiology of Sleep
In cooperation with the sleep laboratory of the ENT hospital (Dr. Traxdorf) we develop methods to objectively identify sleep stages as well as obstructive apnoe events based on EEG data. For this purpose we use a newly in out lab developed technique allowing a statistical comparison of spatio-temporal patterns in the brain. Our aim is for example the objective quantification of the therapeutic success of the CPAP (Continuous Positive Airway Pressure) therapy.
Artificial Intelligence as a tool for the analysis of and as a model for information processing in the brain
This project takes place in cooperation with the Cognitive Computational Neuroscience Group at the Chair of English Linguistics at the intersection of Artificial Intelligence (AI) and Neuroscience. On the one hand, artificial intelligence is used as a tool for the analysis and visualization of high-dimensional data of brain activity of humans and animals, e.g. with imaging methods (EEG, MEG, fMRT), or by electrodes implanted directly into the brain. On the other hand, artificial neural networks, on which all spectacular successes of AI in recent years are based, are excellently suited as models for information processing in the brain. Thus, AI contributes significantly to a deeper understanding of the functioning of the human brain. Not only brain research benefits from this interaction of neuroscience and AI, but AI systems can also become even more efficient by implementing neuroscientific principles of information processing.
Language processing in artificial neural networks and the human brain.
In cooperation with the Cognitive Computational Neuroscience Group at the Chair of English Linguisitics, this project at the intersection of neuroscience, linguistics and artificial intelligence (AI) aims to gain a mechanistic understanding how language processing is implemented in the human brain. This will be achieved by constructing neurobiologically plausible computational and AI models for linguistic tasks which will be tested using behavioral and brain data (questionnaires, MEG/EEG, fMRI). The gained advances during the project - algorithmically inspired by neuroscience - will provide a decisive step towards a framework for robust and flexible natural language processing, being both essential for understanding fundamental principles of intelligent behavior and of potentially great technological value.
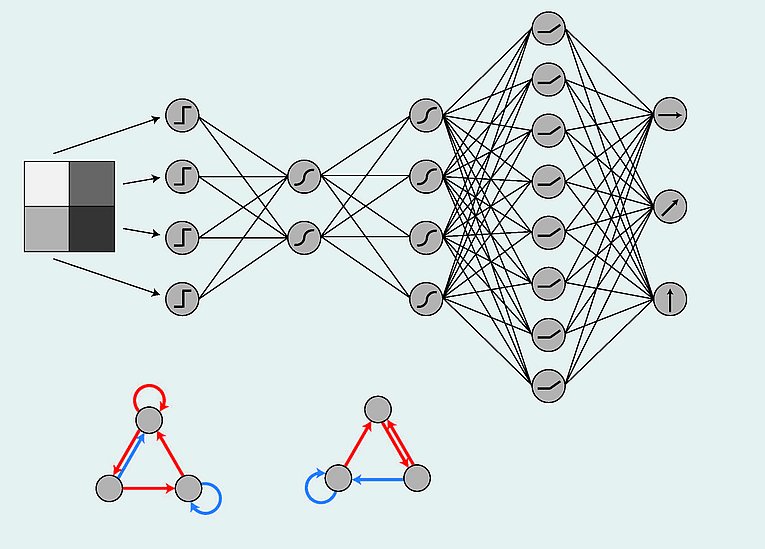
Computational Neuroscience and Theoretical Neurophysics
In this project, together with the Cognitive Computational Neuroscience Group at the Chair of English Linguisitics, we apply the approaches and methods of physics to study (simulated) neural systems and circuits. We aim to analyze, simulate and model fundamental principles of such networks and therefore brain function to understand the relation between neural structure and dynamics. In contrast to common machine learning approaches, we here do not try to optimize a given classification or prediction task, but rather use artificial neural networks as a tool to study the dynamics and rules of (unsupervised) learning. We therefore use methods and concepts from the fields of statistical mechanics, theory of complex systems, graph and network theory, information theory, dynamical systems and chaos theory. The ultimate goal of this project is to describe the minimum set of features of an information processing system (artificial and natural) that are necessary and sufficient for perception, cognition and motor control.